서론
가와사키병의 진단은 주요 임상 소견의 출현 개수에 의존하며, 단일한 확진 진단 검사는 없다[1]. 심장 초음파 검사 결과 발견된 관상동맥 합병증의 존재는 진단적 범주에 포함될 수 있는 것으로 채택된 검사 결과이며[2], 가와사키병의 장기 예후에 가장 중요한 요소이기도 하다. 현재 면역 글로불린의 투여는 급성기에 가장 먼저 투여 되는 효과적인 약제이나[1,3,4], 이의 사용이 일반화된 현재에 있어서도 관상동맥류의 발생 빈도는 2% 내외로 보고되고 있다[5,6]. 또한 면역 글로불린 투여에도 불구하고 발열이 지속되는 저항성 출현은 심한 발병의 증거이며 좋지 않은 예후를 시사하는 소견이다[2]. 따라서, 치료 전에 저항성 출현을 예측해 보고자 하는 다수의 연구 발표들이 있어 왔으나[7–12], 전세계적인 공감대를 형성한 예측 모델은 아직 없는 실정이다[1].
근래 들어, 가와사키병 환자들의 진단과 치료 및 예후에 관련된 주요 논점들에 대하여 기계 학습을 이용한 분석의 모색들이 있어 왔다. 본 논문에서 이러한 연구발표들을 유형별로 정리하여 제시해 보고자 한다.
본론
기계 학습은 인공 지능의 하위 분야라고 할 수 있다. 기계 학습은 광범위한 인간 활동의 분야에서 패턴의 인식, 예측, 분류 및 군집화와 같은 작업을 수행하는 모델을 정립하는 기회를 제공하게 할 수 있다[13]. 기계 학습은 종속 변수(반응 변수)의 유무에 따라 지도 학습과 비지도 학습으로 나눌 수 있다. 그 외, 강화 학습이나, 준 지도 학습과 같은 유형이 추가 분류로 제시되기도 한다. 지도 학습에서 예측의 목표로 채용되는 종속 변수는 문헌에 따라 목표 변수 혹은 라벨(label)로 칭해지기도 한다. 또한, 예측에 있어서 예측 인자로 활용되는 독립 변수는 설명 변수, 입력 변수, 특징(feature), 예측 변수, 회귀 변수 등으로 불릴 수도 있다. 그리고, 종속 변수가 수치형 변수일 때의 기계 학습은 회귀(regression), 범주형 변수일 때의 기계 학습을 분류(classification)로 칭할 수 있다. 자주 사용되는 기계 학습의 방법들을 Fig. 1에 열거하였다. 통상적으로 흔히 적용되는 유형에 따라 대략적 분류를 한 것이므로, 이해에 주의가 필요하다. 예를 들어, multi-layer perceptron의 경우 은닉층(hidden layer)의 수가 2개 이상인 경우 deep learning이라고 하며, 지도 학습에 최적화된 방법이긴 하나, 적용 유형에 따라 비지도 학습이나 강화 학습에 준하여 사용되기도 한다.
가와사키병의 진단 기준 중 발열을 제외한 주요 임상 소견 5가지의 사진 자료들로 훈련된 합성곱 신경망(convolutional neural network, CNN) 모델을 이용하여, 사진 영상 분석을 통해 양성 여부를 판단하게 하는 연구 결과가 Xu et al.에 의해 발표되었다[14]. 합성곱 신경망은 deep learning의 한 가지 방법으로 영상 자료 처리에 효과적이다[15]. 가와사키병 환자 258명과 대조군 251명의 사진 자료들로 연구가 진행되었다. 주요 임상 소견들의 예측에서 receiver operating characteristic curve 면적(AUC)은 0.79–0.91, 민감도 0.77–0.88, 특이도 0.72–0.95 정도였다. 대상자들의 인종 구성과 불완전형 발병의 점유 부분은 확실하지 않다. 유사한 형태의 연구 자료들이 보다 많이 축적된다면, 주요 임상 소견의 양성 여부를 판정하기 어려운 환자들에서의 진단에 도움이 될 가능성이 높을 것으로 기대된다.
Portman et al.은 50명의 가와사키병 환자들 및 100명의 발열 대조군을 포함한 대상자들에서 얻어진 11개의 biomarkers를 포함한 후보 특징들로 지도 학습을 진행하여 가와사키병을 진단하는 기계 학습 모델을 발표하였다[16]. Least angle regression으로 상대적으로 덜 중요한 후보 특징들을 제거하는 방식의 추가 분석이 진행되어, C-reactive protein, N-terminal prohormone of brain natriuretic peptide, thyroid hormone uptake의 세 가지 biomarkers가 최종 모델의 특징으로 선정되었다. 최종 모델의 AUC 0.92, 민감도 0.86, 특이도 0.86이었다. Biomarkers를 이용한 가와사키병의 진단 모색이란 관점에서, 이와 같은 형태의 연구는 향후로도 여러 저자들에 의한 추가적인 발표가 있을 것으로 기대된다.
가와사키병 환자들의 심장 초음파 영상의 deep learning 분석을 통해 관상동맥 병변을 진단하고자 한 연구들이 근래에 있었다[17,18]. 가와사키병에서 심장 관상동맥 합병증은 장기 관찰 시의 가장 중요한 합병증이지만, 급성기를 포함한 발병 초기에도 관상동맥류로 정의할 수는 없으나 직경 크기의 정상 범위 이내에서의 확장을 포함한 이상 소견들이 있을 수 있는 것으로 생각되고 있는데, 2017 American Heart Association guideline에서 관상동맥 직경 z score 값이 차후 1 이상 감소할 때 급성기에 관상동맥 확장이 있었던 것으로 정의한 것도 이러한 생각이 반영된 것이다[1]. Lee et al.이 관상동맥 이상을 deep learning algorithm을 통해 발견하여 불완전형 발병의 진단을 모색한 연구[17]도 이러한 맥락에서 이해될 수 있다.
Xu et al.은 관상동맥 병변의 악화를 예측하기 위한 모델 구축의 연구발표를 하였다[19]. 모델은, 임상 자료들과 6개의 ultrasomics 특징들을 support vector machine으로 기계 학습한 결과가 조합된 것이다. 최종 모델의 AUC 값은 0.83이었다.
앞서의 관상동맥 심장 초음파 영상 분석 연구들과 별도로, 임상 자료와 biomarkers를 조합한 7가지 특징들에 multilayer perceptron 모델의 적용 학습을 통해 관상동맥 병변 발생을 예측하고자 한 연구도 있다[20]. 민감도, 특이도 및 c-index는 각각 73%, 99%, 0.86%이었다.
근래에 기계 학습을 적용한 면역 글로불린 저항성의 예측에 대한 연구 발표들이 있었으며, 이들에서 적용된 방법들 및 평가 결과들이 Table 1에 제시되어 있다[21–26]. 이 연구들에서 공통적으로 기존의 가장 많이 인용되는 세 가지 통계학적 예측 모델들- Kobayashi score[8], Egami score[7], Sano score[9]에 대한 병행 분석이 진행되었었는데, 새로 개발된 기계 학습 모델의 결과가 더 우수했다는 것으로 보고되고는 있다. 그러나, 가장 처음 수행된 Takeuchi et al.의 연구 발표 이외의 것들은 대체로 민감도가 낮아 보이는데, Takeuchi et al.의 연구는 모델 개발 후 평가 자료 집합(validation set)에서의 검증 단계가 없었던 단점이 있다[21]. 가장 최근에 Lam et al.에 의한 발표[26]에서는 기계 학습 모델의 결과들 조차 아직은 실용적이지 않다는 회의적 견해와 함께 면역 글로불린 저항성의 예측에 임상 검사실 자료들을 사용하는 것 자체에 대한 문제 제기가 있었다. 면역 글로불린 저항성을 라벨로 지도 학습을 통해 모델을 개발한 후 추가로 uniform manifold approximation and projection을 통한 차원 축소 분석을 진행하였다. 독립 변수들 전체가 2차원으로 차원이 축소되어 전체 대상자들을 요약된 두 가지 특징으로 2차원 그래프 상의 점으로 표시할 수 있게 되었던 것인데, 여기서 면역 글로불린 저항성이 전체 자료의 분포에 있어서 아무런 특이 분포 패턴을 보여 주지 못하였던 것이다. 이는 면역 글로불린 저항성이란 변수가 전체 환자들을 이에 따라 충분히 구분하여 예측하는 것에 한계가 있다는 의미로 해석되므로, 이 결과에 근거하여 면역 글로불린 저항성을 예측하기 위하여는 임상 검사 자료들 만으로는 부족하며, 추가적인 biomarkers가 독립 변수로서 필요할 것으로 저자들은 결론 지은 바 있다.
면역 글로불린 저항성의 예측 문제에서 추가로 논의가 필요한 부분은 기계 학습 방식의 내재적인 한계와 관련된 것이다. 지도 기계 학습을 통해 모델을 개발할 경우 예측력을 높일 수는 있겠으나, 특징들이 어떻게 라벨을 예측했는지 알 수 없는 블랙박스와 같은 현상을 보이게 된다. 통계적 방법으로 개발된 모델과 달리 특징들과 라벨 간의 인과 관계 혹은 수치 정량적 관계들을 확인할 수가 없게 되게 된다. Shapley additive explanation(SHAP)은 기계 학습으로 개발된 모델의 라벨 예측에, 특징들이 갖는 중요성을 평가하고자 하는 유력한 방법이다[27,28], 면역 글로불린 저항성 예측에서도 SHAP 분석이 적용된 적이 있다[23,25]. Sunaga et al.은 기계 학습 모델 개발 이후 추가로 SHAP 분석 후 세 가지 변수 - 치료 개시일, C-reactive protein 수치, cholesterol 수치를 추출하여 통계적 모델과 동일한 형태의 예측 모델을 새로 개발하기도 하였다[25]. SHAP 분석 후 결과를 보여주는 그림의 예를 Fig. 2에 제시하였다.
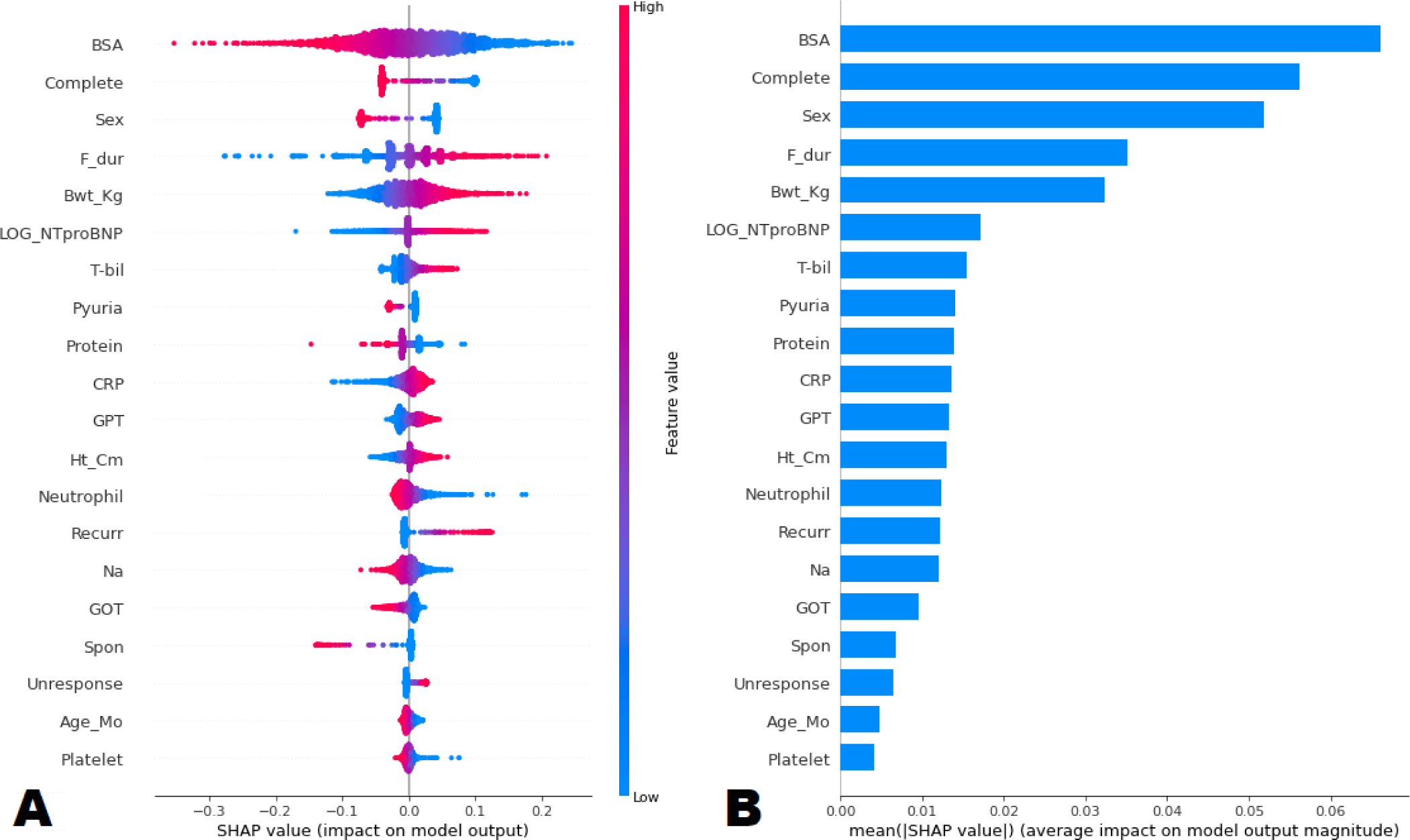
Ghosh et al.은 166개의 gene signature가 모든 viral pandemic에서 나타나며, 이 중 20개의 genes가 심한 염증을 보여주는 것으로 발표한 바 있으며, MIS-C 환자들과 가와사키병 환자들의 비교 분석 결과, 두 가지 질환이 면역 병리학적으로 일부 공통된 병리 기전을 공유하고 있다는 연구 발표를 하였다[29]. 그들이 연구를 진행함에서 여러 단계에서 컴퓨터 기반의 학습 방법들을 적용한 바 있다. 광범위한 자료들 내에서 패턴을 찾거나 그룹을 분류할 필요가 있는 이와 같은 유형의 연구에서 기계학습 방법들의 적용은 향후로도 증가될 것으로 기대되는 바이다.