Introduction
Kawasaki disease (KD) is an acute pediatric disease of unknown etiology that primarily affects East Asia [1,2]. If not treated promptly, about 25 percent of KD patients develop coronary artery aneurysms (CAA) which can lead to thrombosis, myocardial infarction, or ischemic heart disease [1,2]. Patients with CAA require long-term follow-up and special care. The occurrence of major patient outcomes in CAA is unpredictable, and currently, there is no predictive metric to guide clinical decisions [3-8]. The American Heart Association (AHA) treatment guidelines for KD classify the aneurysm risk based on the size of the aneurysm [1]. However, coronary artery disease and thrombosis are associated not only with the vessel geometry but also with abnormal hemodynamics and the biological processes in the diseased artery [1]. Therefore, a multifactorial approach is necessary for advanced treatment planning of KD patients. Recent treatment approaches for KD patients have started incorporating multi-modality tools and methodologies to identify the disease progression mechanisms to prevent major clinical outcomes. Among those, the computational fluid dynamics (CFD) is being increasingly used in clinical decision-making, risk assessment, surgery planning, and medical device design for the treatment of pediatric cardiovascular disease [3-8]. Several CFD studies have investigated the effect of blood flow dynamics on the major clinical outcomes of CAA in KD patients to guide the medical treatment.
In the next sections, I will review previous studies that have used CFD to investigate the hemodynamics in CAA caused by KD and evaluate the potential of the CFD technique to improve the treatment guidelines of KD. I will elaborate on methods used in the CFD modeling, simulation, and post-processing of image-based data. I will also summarize key findings on hemodynamics and their clinical significance. Finally, I will address the limitations of previous studies and propose potential avenues for future research using CFD in the context of KD.
Main Subject
The patient-specific CFD approach incorporates the medical images and clinical data from each patient to simulate blood flows in CAA. The patient-specific CFD uses CT/MRI images to construct a 3D anatomic model of the coronary arteries and a part of the aorta (Fig. 1). To perform CFD, conditions at the boundaries of the three-dimensional model (boundary conditions, BC) are specified in the form of numerical values of flow, pressure, wall rigidity (modulus of elasticity), or vascular resistance. Blood flow information can be directly measured from MRI, echocardiography, or invasive tools and then prescribed at the inlet of the aorta as a BC. Rule-based morphometry relationships derived from population studies are used to prescribe the BC at the outlet of the computational model connected to the peripheral arteries. Lumped parameter network (LPN) models consisting of resistances and capacitances are tuned to reproduce the physiologic response of the downstream hemodynamics connected to the outlet of the model. The 3D anatomical model with fully prescribed BC is then used to simulate the blood flows by solving the mathematical equation that governs the dynamic of blood flows - the Navier-Stokes and continuity equations. Three-dimensional flow and pressure fields inside the patient-specific anatomical model are obtained after numerically solving the governing equations. These detailed flow and pressure fields are post-processed to compute hemodynamics quantities associated with the cardiovascular disease progression non-invasively.
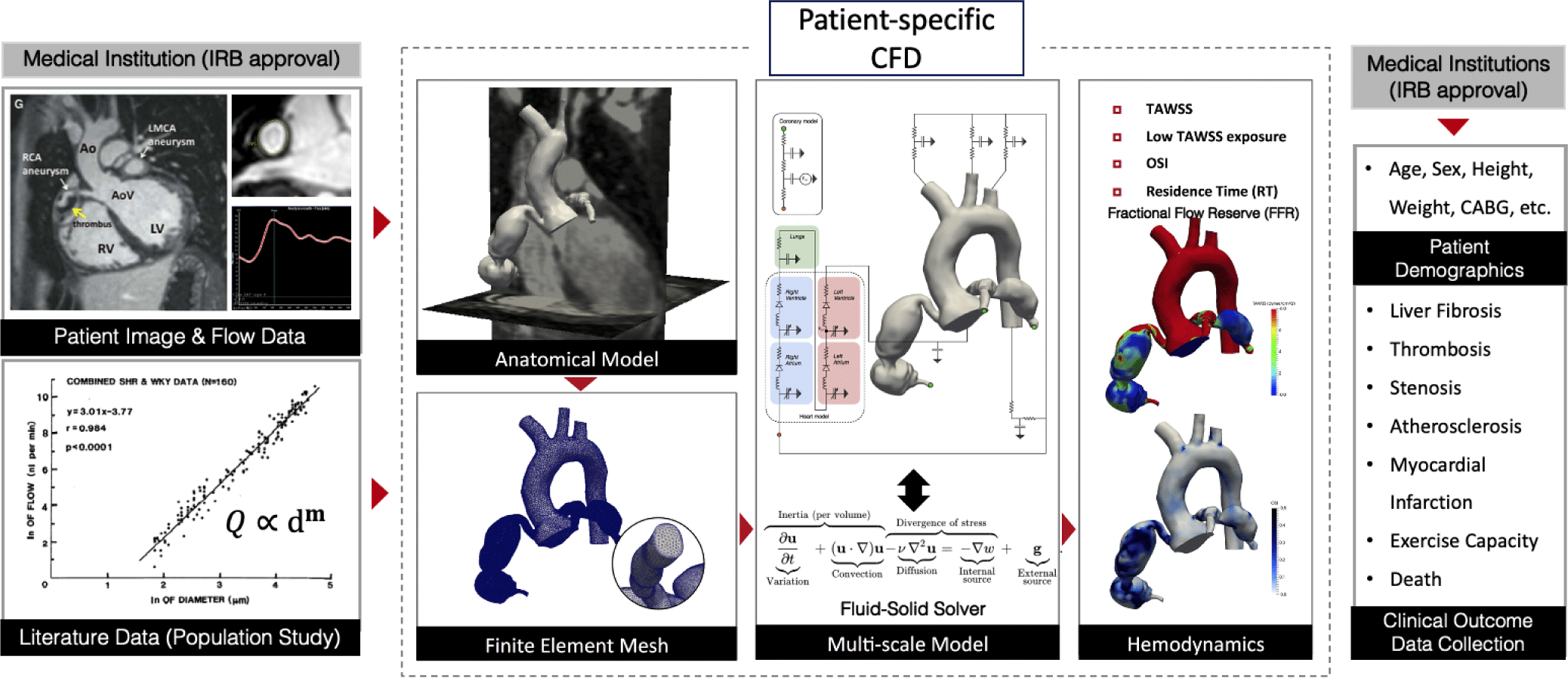
Sengupta et al. [4] is the first study that applied the patient-specific CFD to investigate the hemodynamics in CAA caused by KD (Table 1). They performed a hemodynamics simulation on a three-dimensional anatomical model of the coronary arteries and aorta from a 10-year-old subject who had suffered from KD at the age of 3 years. This foundational CFD study applied the computational framework from adult coronary artery simulations [3] to a patient with KD and quantified the hemodynamics in the CAA, including velocity, time-averaged wall shear stress (TAWSS), oscillatory shear index (OSI), and particle residence time (RT, also known as CET) [4].
Study | Patient country | Age at KD | N | Image | Aneurysm geometry | CFD-derived hemodynamics | Clinical outcomes |
---|---|---|---|---|---|---|---|
Sengupta et al. [4] | USA | 3 | 1 | CT | Z-score, Sphericity | Velocity, TAWSS, WSSG, CET, OSI | Thrombosis |
Sengupta et al. [5] | USA, Russia | 1−16 | 6 | CT | Z-score, Sphericity | Velocity, TAWSS, OSI, PRTg | Thrombosis |
Grande et al. [6] | Canada | 0−12 | 10 | MRI | Z-score, Dmax, AWSS, Sa | Velocity, TAWSS, WSSG, OSI, RT | Thrombosis |
Grande et al. [7] | Canada | N/A | 6 | MRI | Not discussed | AP, PolyP | Thrombosis |
Menon et al. [8] | Japan, USA | N/A | 15 | CT | Z-score, Dmax/Dmin | TAWSS, RT, FFR | Myocardial ischemia |
AP: activated platelet; CET: cumulative exposure time; CFD: computational fluid dynamics; CT: computed tomography; Dmax: maximum aneurysm diameter; Dmin: minimum diameter; FFR: fractional flow reserve; MRI: magnetic resonance imaging; N: sample size; OSI: oscillatory shear index; PolyP: polyphosphate; PRTg: particle residence time gradient; RT: residence time; Sa: aneurysm surface area; WSS: time-averaged wall shear stress; WSSG: wall shear stress gradient; N/A: not available.
TAWSS and OSI are well-known hemodynamic parameters that are correlated with endothelial cell dysfunction. Particle residence time is a hemodynamic index that measures how long blood flow remains in the same region. It is calculated by tracking the time of computationally seeded particles advected by the blood flow [9]. RT is known to be associated with the formation of thrombosis. Using the computational model, Sengupta et al. virtually manipulated the CAA to a normal size coronary artery and compared the “virtually healed” model (normal control) with the real patient data (with CAA) [4]. A major finding of this study was the measurement of a high particle residence time of 5 cardiac cycles in the CAA due to flow recirculation, whereas normal coronary arteries had a particle residence time of less than 1.3 cycles, allowing particles to wash away immediately. The study used a simple computational modeling strategy such as the rigid vessel wall assumption and open-loop boundary conditions for flow circulation in coronary artery beds with an assumed intramyocardial pressure. This pilot study pioneered CFD research on KD and demonstrated the potential of the CFD technique for further studies on KD-associated CAA.
A subsequent study by Sengupta et al. was the first CFD study to retrospectively collect clinical outcome data from KD patients and relate it to hemodynamic variables [5]. They expanded on the scope of previous research by recruiting six KD patients and collecting clinical information on medical therapy, calcification, thrombosis, and surgery. Among the eleven CAAs studied, the authors distinguished five with thrombosis and six without thrombosis. They found that the time-averaged wall shear stress (TAWSS) was low, and the particle residence time (RT) was high in the CAAs with thrombosis compared to the control arteries.
While the geometric indices of CAA, such as the maximum aneurysmal diameter, aneurysm sphericity, and aneurysm shape index, were not able to clearly distinguish the occurrence of thrombosis in CAA, hemodynamic parameters derived from CFD, such as TAWSS and particle residence time, were better able to predict the thrombotic risk. In this study, a deformable wall model was used in the computational model to capture vessel compliance via a fluid-structure interaction framework. The coronary boundary condition used an offline lumped parameter network (LPN) heart model to obtain the intramyocardial pressure. These computational treatments were more advanced methodologies that better reproduced the realistic, patient-specific physiologic response of the blood flow and vessel wall compared to the previous study.
The next CFD study, performed by Grande et al., increased the sample size to 10 patients and further improved computational modeling methodologies [6]. Among the ten patients, five developed thrombosis in their CAA. Statistical analysis on a total of 32 aneurysms (5 thrombosed) was performed. This study revealed significant differences between CAAs without thrombosis and those with confirmed thrombosis when hemodynamic variables (TAWSS, RT) were used. The performance of hemodynamic variables was superior to that of geometric parameters (aneurysm surface area, maximum diameter, and Z-score) [10]. The authors did not find significant differences between thrombotic and non-thrombotic branches based on geometric parameters. Receiver operating characteristics (ROC) analysis on the hemodynamic parameters revealed that RT > 2 cycles was the best predictor for thrombotic events, with an area under the ROC curve of 0.92, sensitivity of 1, and specificity of 0.73. In this study, computational approaches were also improved compared to previous studies. The elasticity of the deformable vessel wall was supplemented by time-series imaging data within a heart cycle. The closed-loop LPN heart model was fully coupled to the three-dimensional model and run online. The LPN values were automatically tuned to match patient-specific clinical data. This study demonstrated the viability of CFD techniques for clinical decision-making for the treatment of KD with a cutoff derived from statistical analysis.
Later, Grande et al. presented an advanced computational model that integrated the hemodynamics with the chemical reaction and the platelet adhesion [7]. All previous studies solely relied on hemodynamics but did not consider the interaction between biological process and hemodynamics. To incorporate the complex biological processes and chemical reactions in the computational model, Grande et al. coupled the chain reactions related to the thrombosis with the mechanical factors derived from CFD [7]. Using this model, the authors found that the region of thrombosis was matched with the biochemical activation of the pro-coagulant measured by activated platelets, local concentrations of Adenosine Diphosphate, and Polyphosphate. This pilot study used six patient data on the same patient group data from Grande et al. [6].
Most recently, Menon et al. conducted a study to evaluate the risk of myocardial ischemia on CAA by using CFD to compute fractional flow reserve (FFR) [8]. This study is unique in that it is the first to focus on myocardial ischemia rather than thrombosis in CAA. The authors collected 15 KD patients with aneurysms and investigated 153 coronary arteries for statistical analysis. They obtained FFR, which was the golden standard metric for assessing the functional significance of coronary artery anomalies [11]. The authors found FFR showed a weak correlation with aneurysm Z-scores but correlated better with the ratio of the maximum lumen diameter with aneurysms to minimum lumen diameter to aneurysms. The pressure drop induced by the CAA did not lead to FFR values below the cutoff indicating functional significance (< 0.8) [11]. This result agreed with the findings from the invasive measurement study using a catheter [12]. Furthermore, the study confirmed the consistent conclusion from previous CFD studies that the maximum diameter or Z-score were not able to clearly stratify the risk of coronary artery disease in KD patients. However, this study only used KD patient images with aneurysms and did not include stenotic arteries in their analysis. This study was the first to investigate FFRCT for pediatric patients, particularly for KD, and expanded the research scope beyond thrombosis.
The AHA guideline classified the risk of coronary artery abnormalities during follow-up based on the geometric parameters: Z-score and maximum diameter. The guideline classified CAA into; 1: No involvement (Z-score < 2), 2: dilation only (Z-score 2 to < 2.5), 3: small aneurysm (Z-score ≥ 2.5 to < 5), 4: medium aneurysm (Z-score ≥ 5 to < 10, and absolute dimension < 8 mm), 5: large or giant aneurysm (Z-score ≥ 10, or absolute dimension ≥ 8 mm) [1]. The remodeling of the aneurysm further divided these categories into subcategories based on whether CAA persists or regresses. Medical therapy algorithm was suggested based on this classification. However, the clinical outcomes of KD patients are highly unpredictable. In practice, clinicians often rely on their experience and protocol instead of following the guideline. The current treatment guideline may benefit from more comprehensive analysis including the hemodynamic analysis combined with the geometry of the aneurysm, which has been shown to be a better predictor of the clinical outcome.
The coronary artery aneurysm causes an abnormal blood flow pattern - flow recirculation. In the recirculating region, blood flow is trapped inside the aneurysm for a longer period than in a normal coronary artery. Additionally, the three-dimensional high velocity gradients in the recirculating zone contribute to the total energy dissipation, which results in a pressure drop. This flow phenomenon leads to the significant quantitative differences in hemodynamics metrics between thrombotic and non-thrombotic branches. From the reviewed CFD studies, consistent conclusions can be drawn about the hemodynamic metrics in CAA. First, the time-averaged wall shear stress (TAWSS) is significantly lower in the coronary artery with thrombotic aneurysms compared to the non-thrombotic coronary arteries. Second, the particle residence time is higher in the aneurysms with thrombosis [5,6]. Third, the blood pressure drops across the upstream and downstream of the aneurysm are larger than the pressure drop through the normal coronary arteries [8].
Grande et al. demonstrated that the diagnostic performance of the CFD-derived hemodynamics (WSS and RT) was superior against the geometry-based metrics primarily used in current AHA guidelines [6]. They also showed that the residence time and the low wall-shear stress area are the better predictor of thrombosis. Menon et al. reported that the fractional flow reserve was not correlated with the aneurysm diameter [8]. They found that the ratio between minimum and maximum diameter was a better predictor of FFR drop.
All reviewed studies used the open-source software Simvascular for computational modeling and hemodynamics simulation [13]. Simvascular implements the variational multiscale finite element method for discretization of the Navier-Stokes equation (momentum conservation) and continuity equation (mass conservation). The validity of Simvascular has been thoroughly tested and compared against analytical solutions in an idealized geometry, CFD results from different solvers and groups on an aneurysm model [14], and in vitro phantom studies [15,16]. Simvascular’s predictive capability has been demonstrated against in vivo data through invasive measurements in other disease applications [17,18].
One of the most relevant validation efforts to KD is an experimental study by Kung et al. [15]. Kung et al. created a physical phantom model reconstructed from the three-dimensional computational model of KD-CAA and measured the hemodynamics inside using PC-MRI. Their in-vitro experimental setup consisted of a closed-loop flow system and a computer-programmable pulsatile pump that produced the resting and exercise flow waveform. The results showed good agreement between the velocity fields from CFD results and those from the experiments, with errors between 5%−17%. This study confirmed the accuracy of CFD and demonstrated the viability of CFD for the non-invasive measurement of hemodynamics for KD patients.
The major limitation of the previous CFD study is the small sample size. Even in the largest study by Menon et al., the number of patients was only 15 [8]. As first pointed out by Sengupta et al., a substantially larger cohort (at least 100) is required to demonstrate statistical significance in comparisons between patients with and without thrombus [5].
Furthermore, previous studies used mixed imaging modalities, which hindered the integration of clinical implications obtained from different studies. Sengupta et al. [4,5] used CT images to reconstruct the anatomic model, whereas Grande et al. [6,7] used MRI. This lack of consistency may be due to the availability of patient data.
Also, all previous CFD research performed cohort analyses on CT images at a particular time of disease after KD diagnostics. In the current computational framework, CFD provides hemodynamic information at the time of CT, however, it is not able to predict the long-term vascular remodeling of the CAA. It is under question whether the currently suggested hemodynamic variables are correlated with the disease progression in time. Persistency or regression of CAA is an important criterion for the risk stratification [1]; therefore, a time-series analysis of KD CAA must be performed to identify causes of the vessel growth or regression.
In addition, although thrombosis is a major adverse outcome in KD patients, there are other important clinical outcomes in KD patients. Other major clinical outcomes of interest include myocardial ischemia, (sudden) death, and calcifications. While Menon et al. started giving attention to the FFR in coronary artery aneurysms, the study only included the patients with aneurysms [8]. Clinical observations indicate that stenosis may also occur in KD patients, and they often form at regions near the aneurysms. In this case, the contribution of pressure drops due to stenosis and aneurysm to the functional significance of coronary artery should be quantified, as first pointed out by Grande et al. [7].
Lastly, a major drawback of the CFD technique in the application to clinical settings is the high computational modeling and simulation cost. Currently, a standard three-dimensional coronary artery CFD simulation takes roughly 4−8 hours on multi-core supercomputing clusters. Fluid-structure interaction and the closed-loop LPN model adds more computational complexity and cost by a factor of two or more. Furthermore, creating a three-dimensional anatomic model from image data is a laborious process, which can set a bottleneck for large cohort studies as well as the application of CFD technology in a clinical timeline.
First and foremost, a large cohort study with a sufficient sample size, possibly over 100 KD patients, should be conducted to confirm the statistical significance of the previously identified hemodynamic metrics and their association with clinical outcomes. Recruitment of such a large number of KD patients is challenging due to the limited availability of CT images and clinical data. This study is likely to be planned in East Asian countries, such as South Korea and Japan, where KD is more prevalent. If the large cohort study confirms the association between the hemodynamic variables and clinical outcomes, a prospective study could follow. This study would require multidisciplinary collaboration among pediatric cardiologists, radiologists, and mechanical engineers to ensure proper clinical and technical expertise and resources.
Time-series analysis may reveal the association between hemodynamic parameters with the growth and remodeling (G&R) of the CAA in KD patients. Currently, the progression or regression of CAA is poorly understood, and there is no metric related to the vessel wall remodeling. To understand the G&R, multiple snapshots of the anatomical features of CAA (via CT/MRI) and clinical information must be stored through a long-term follow-up. Once such data are collected, hemodynamic factors associated with the progression or regression can be identified.
For the clinical acquisition of CFD technology, a reduced-order modeling technology should be developed to deliver hemodynamic evaluations in a clinically available timeline. There are available reduced-order modeling tools in Simvascular, which reduce the three-dimensional anatomic model to one-dimensional or zero-dimensional (i.e., LPN) models with much lower computational cost. Application of this reduced order modeling tool can accelerate the simulation process when they are optimized to accurately predict the hemodynamics of the three-dimensional model. The surrogate models will enable the assessment of the credibility of predictions via an uncertainty quantification framework [19].
Conclusion
The etiology of the KD is still unknown. Current guideline for KD does not reflect a comprehensive understanding of hemodynamics and heavily relies on the size of the aneurysm. CFD can provide detailed hemodynamic evaluations within the aneurysms. Reviewed retrospective CFD studies in this paper showed promising results for the risk assessment of KD CAA based on hemodynamic variables. Consistently, the patient clinical outcomes were less correlated with the geometric parameters alone, suggesting future guidelines of KD based on hemodynamics analysis. One of the major drawbacks of previous studies is the limited number of included KD patients. A large-cohort study should follow to verify these findings. For clinical integration of this CFD technology, the cost of the patient-specific modeling and CFD simulation should be reduced via surrogate models.